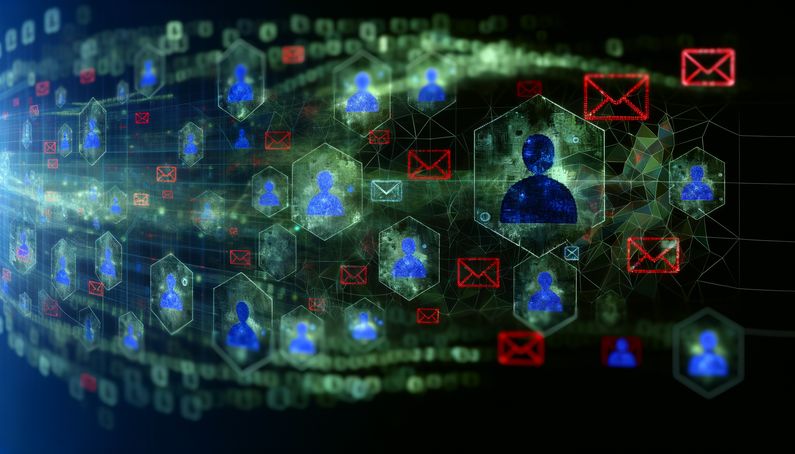
Navigating the Challenges of Machine Learning in Email Security
Machine learning (ML) has become a cornerstone in enhancing email security, yet it is not without its challenges. A recent incident involving Microsoft Exchange Online highlights the complexities ML models face in distinguishing legitimate emails from spam. Users experienced disruptions when emails from Gmail accounts were mistakenly flagged as spam, illustrating the potential pitfalls of ML misclassification (Bleeping Computer). This incident underscores the need for continuous adaptation of ML models to keep pace with evolving cyber threats and maintain a balance between security and usability. As cyber threats become more sophisticated, the integration of ML with existing security protocols and the ability to detect threats in real-time are crucial for effective email security.
Machine Learning Challenges in Email Security
Misclassification of Legitimate Emails as Spam
One of the significant challenges in email security using machine learning (ML) is the misclassification of legitimate emails as spam. This issue was notably observed when Microsoft Exchange Online’s ML model mistakenly flagged emails from Gmail accounts as spam, impacting users by automatically moving these emails to the junk folder (Bleeping Computer). This misclassification can occur due to the ML model’s inability to accurately distinguish between legitimate emails and those that resemble spam attacks. The challenge lies in the model’s reliance on patterns and similarities that can sometimes lead to false positives, affecting communication and productivity.
Continuous Adaptation to Evolving Threats
Machine learning models in email security must continuously adapt to the rapidly evolving landscape of cyber threats. As threat actors develop new phishing techniques and spam methods, ML models must be updated to recognize these novel patterns. The complexity of this challenge is highlighted by the need for models to learn from vast amounts of data and adjust their algorithms accordingly. For instance, Microsoft’s Exchange Online has faced multiple instances where its ML models required adjustments due to evolving spam tactics, such as the incident in October 2023 where a bad anti-spam rule had to be disabled to prevent flooding of admins’ inboxes with BCC emails (Bleeping Computer).
Balancing Security and Usability
Another challenge in implementing ML in email security is maintaining a balance between security and usability. While stringent security measures are necessary to protect against cyber threats, they can sometimes lead to usability issues, such as legitimate emails being quarantined or blocked. This balance is crucial to ensure that users can efficiently communicate without unnecessary disruptions. For example, in March 2024, Microsoft confirmed that outgoing emails were being rejected as spam, causing significant inconvenience to users and administrators (Born’s Tech and Windows World).
Integration with Existing Security Protocols
Integrating ML models with existing email security protocols presents another challenge. Email security systems often rely on established protocols such as SPF (Sender Policy Framework) and DKIM (DomainKeys Identified Mail) for authentication. ML models must work in conjunction with these protocols to enhance security without causing conflicts or redundancy. For instance, Google’s introduction of stricter rules for bulk emails required the integration of ML models with these protocols to ensure compliance and prevent legitimate emails from being marked as spam (The Register).
Real-time Threat Detection and Response
The ability to detect and respond to threats in real-time is a critical challenge for ML models in email security. Cyber threats, such as phishing attacks, can cause significant damage if not addressed promptly. ML models must process and analyze incoming data quickly to identify potential threats and take appropriate action. This challenge is compounded by the need for high accuracy in threat detection to minimize false positives and negatives. AI and ML technologies are pivotal in achieving real-time threat detection, as they can analyze vast amounts of data and identify patterns that human operators might miss (Acronis).
In summary, while machine learning offers significant advantages in enhancing email security, it also presents several challenges that need to be addressed. These challenges include misclassification of legitimate emails, continuous adaptation to evolving threats, balancing security and usability, integration with existing protocols, and real-time threat detection and response. Addressing these challenges is essential to maximize the effectiveness of ML models in protecting against cyber threats.
Final Thoughts
The journey of integrating machine learning into email security is fraught with challenges, yet it holds immense potential for enhancing protection against cyber threats. The Microsoft Exchange Online incident serves as a reminder of the delicate balance required between security and usability. Continuous adaptation and integration with existing protocols are essential to address the evolving landscape of cyber threats. As we move forward, the focus must remain on refining ML models to minimize misclassification and enhance real-time threat detection, ensuring that email communication remains both secure and efficient (Bleeping Computer).
References
- Bleeping Computer. (2023). Microsoft fixes Exchange Online bug flagging Gmail emails as spam. https://www.bleepingcomputer.com/news/microsoft/microsoft-fixes-exchange-online-bug-flagging-gmail-emails-as-spam/
- Born’s Tech and Windows World. (2024). Exchange Online: Microsoft bestätigt Blockade ausgehender Mails als Spam März 2024. https://www.borncity.com/win/2024/03/10/exchange-online-microsoft-bestatigt-blockade-ausgehender-mails-als-spam-marz-2024/
- The Register. (2024). Microsoft external recipient limit. https://www.theregister.com/2024/04/16/microsoft_external_recipient_limit/
- Acronis. (n.d.). AI in email security. https://www.acronis.com/en-us/blog/posts/ai-email-security/)